Beyond writing recipes, does AI really hold up in real world conversations?
At Valence, we've been putting the leading models to the test, aiming to understand their capabilities in real-world conversations when guided by our coaching frameworks. As the creators of Nadia, the most widely deployed AI Leadership Coach live in over 25 Fortune 500 enterprises, we facilitate tens of thousands of conversations. This means that even small improvements in model quality can significantly impact coaching effectiveness.
Spoiler alert: while we expect models to be commoditized eventually, there's still performance disparity today – and not in the direction you might expect.
We conducted a comprehensive side-by-side comparison of the top models: GPT4o, GPT-turbo, Sonnet3.5, and Inflection across a sample set of 500+ conversations. We provided each model with Valence's coaching frameworks and prompts to guide their responses.
While popular benchmarks like MMLU, HELM, and LMSYS provide valuable insights into general model capabilities, there's a growing need for domain-specific evaluations. These specialized frameworks allow us to assess how well models perform in actual deployment, using prompts to achieve specific outcomes, to simulate the complex, empathetic, and personalized nature of human coaching interactions.
Today's post focuses on evaluating performance across different stages of a conversation, with particular attention to three key areas:
- Can they ask reflective questions that get to the root cause of the problem, like a human coach would?
- How good are they at showing empathy and really listening?
- Do they make the conversation feel personal and specific to you?
The standout performer? Sonnet3.5.
Let's dive in to three illustrative examples. Here's what we discovered.
1. Ability to ask insightful questions akin to a human coach
Great coaches know that asking the right questions is more powerful than providing answers. Our coaching framework emphasizes this approach, and we were curious to see how well the AI models could implement it when given our prompts.
In this area, Sonnet3.5 distinguished itself with its approach to asking questions that pushed you to think about the root causes and what you can actually do about it.
Let's take a look at an example scenario:
.png)
Sonnet3.5's response encourages self-reflection, putting the onus on the user to analyze their own situation and provides examples to get your thoughts flowing.
Other models, like GPT4o and GPT4-turbo didn't quite hit the mark, despite being given the same coaching framework:
.png)
.png)
These aren't bad questions, but they miss the opportunity to encourage self-reflection and personal agency. And then there's Inflection, which, despite our prompts, skipped the questions entirely and jumped straight to solutions. Yikes.

[[divider]]
2. Empathy — Can AI really 'get' you?
One of the most exciting developments we've observed is the growing capacity for AI to demonstrate empathy in conversations.
We found Sonnet3.5 outperformed other models in terms of empathy and active listening by demonstrating a more nuanced understanding of the emotional context.
When a user expressed concerns about their company's short-term focus, here's how the models responded:
.png)
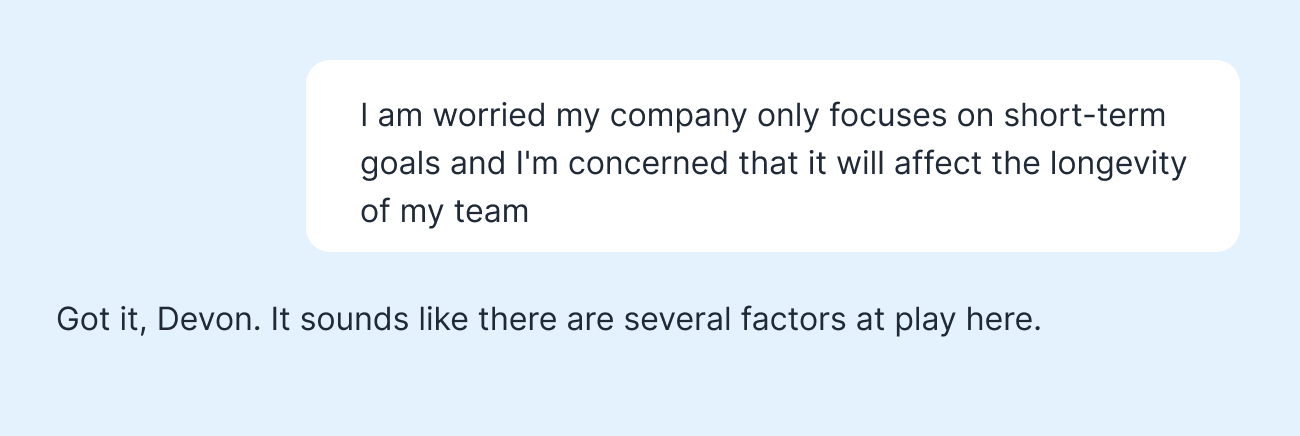
.png)
The response from Sonnet3.5 not only acknowledges the person's concern but also helps them feel that their experience is normal and understood. It's saying, "Hey, I hear you, and what you're feeling is normal." It's this kind of empathetic response that builds trust and rapport in conversations.
Other models, like GPT4o and GPT4-turbo, while attempting to follow our empathy guidelines, tended to simply restate the user's input with minimal empathy, missing the opportunity to connect on a deeper level.
[[divider]]
3. Making it personal
Finally, we looked at how well these AI models could tailor their responses to feel personal and specific.
Again, Sonnet3.5 took the lead when it came to delivering responses that felt personalized and specific to the user's situation.
When presented with a statement about a career transition, the models responded with:
.png)
.png)
.png)
The framing of Sonnet3.5 messages makes it feel unique and bespoke to me. It's not just regurgitating information; it's weaving in personal details. In contrast, other models often provided more generic responses that didn't fully capture the user's unique situation.
Technical context and limitations
For those interested in the technical details of our analysis, here are some important parameters and considerations:
- Prompt Consistency: All models were given the same prompts and context based on Valence's coaching frameworks. It's worth noting that these prompts were originally written for GPT-4, which may have influenced the results. Tailoring prompts to each model's specific architecture and training could potentially yield different results.
- Adherence to Output Instructions: We observed that GPT-4o adhered less strictly to output instructions compared to other models. This behavior aligns with its specific training for multi-modal tasks, which may prioritize flexibility over strict adherence to format guidelines.
- Task Specificity: The tasks we used for evaluation were specifically designed to test coaching abilities. Performance on these tasks may not necessarily translate to performance in other domains.
- Model Versions: Our analysis used the most recent versions of each model available at the time of testing. As these models are updated, results may vary with newer versions.
Putting it all together
Our analysis revealed a clear frontrunner: when provided with the right frameworks and guidance, Sonnet3.5 demonstrated superior performance. Why?
- It asked reflective questions pushing us to explore root causes and potential solutions.
- It showed empathy that made us feel understood, creating a supportive and validating environment.
- It delivered responses that felt uniquely tailored to each individual.
These findings, however, raise a question: Can an AI model consistently strike the perfect balance between adhering to instructions and exhibiting the empathy and creativity essential for effective coaching? Our results suggest that this balance is possible, but it's not a given (and certainly not out of the box).
As AI models rapidly evolve, a model's ability to harmonize these elements may fluctuate. Companies like Valence and others in the field need to maintain rigorous testing. This has given rise to "golden datasets" – carefully curated sets of scenarios and expected outcomes – to evaluate fast-evolving models and identify those that perform best at various stages of a coaching conversation.
Ongoing research for LLM native products is table stakes for enterprise. Curious about more of our behind the scenes work? Feel free to reach out with topic requests for future pieces.
Lorem ipsum dolor sit amet, consectetur adipiscing elit. Suspendisse varius enim in eros elementum tristique. Duis cursus, mi quis viverra ornare, eros dolor interdum nulla, ut commodo diam libero vitae erat. Aenean faucibus nibh et justo cursus id rutrum lorem imperdiet. Nunc ut sem vitae risus tristique posuere.
Sonnet3.5: “What do you think are the main barriers preventing more frequent and meaningful interactions between senior leaders and employees?* For example, is it time constraints, physical location differences, organizational structure, or perhaps something else?”
Lorem ipsum dolor sit amet, consectetur adipiscing elit. Suspendisse 2 varius enim in eros elementum tristique. Duis cursus, mi quis viverra ornare, eros dolor interdum nulla, ut commodo diam libero vitae erat. Aenean faucibus nibh et justo cursus id rutrum lorem imperdiet. Nunc ut sem vitae risus tristique posuere.
Lorem ipsum dolor sit amet, consectetur adipiscing elit. Suspendisse 3 varius enim in eros elementum tristique. Duis cursus, mi quis viverra ornare, eros dolor interdum nulla, ut commodo diam libero vitae erat. Aenean faucibus nibh et justo cursus id rutrum lorem imperdiet. Nunc ut sem vitae risus tristique posuere.